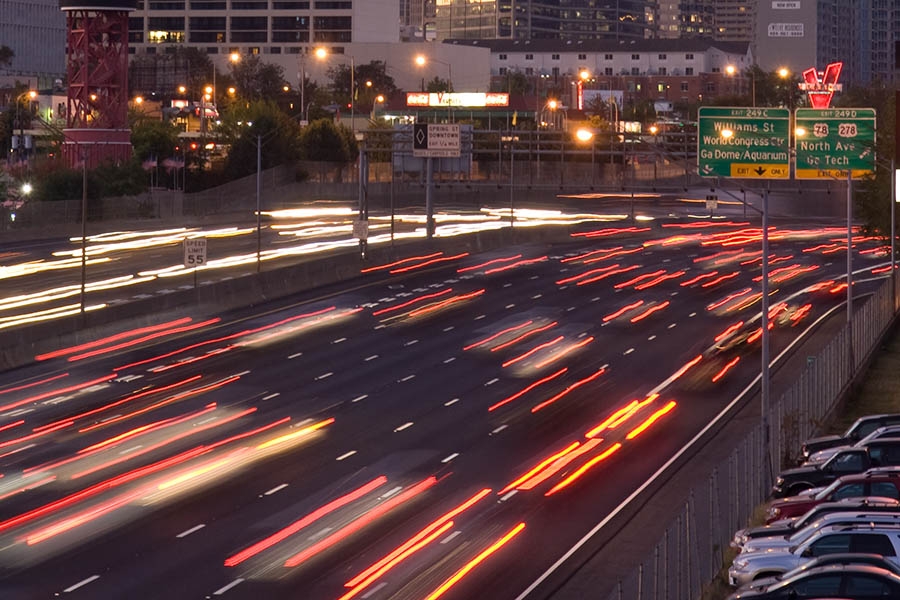
![]() Cars speed along the Interstate 75/Interstate 85 Downtown Connector in Atlanta. Assistant Professor Samuel Coogan will spend the next five years building the mathematical foundation for models and techniques that will offer new ways to control traffic flow on roads like this one. With the support of a National Science Foundation Early Career Development award, Coogan will use the mountains of new data available about our transportation networks to build his models and then prove that they work. (Photo: Rob Felt) |
The day is coming — perhaps not even that far into the future — when most cars on the road will communicate with each other. They’ll share information with the traffic signals and ramp meters they encounter. Some of those cars will be driving themselves.
Roads will be packed with sensors. And wireless technology means we’ll be able to collect mountains of data every second in real time.
Couple all of that with the reality that we’ve more or less maxed out our roadway infrastructure and the result is what Samuel Coogan calls an unprecedented opportunity to reshape how we operate our transportation systems.
![]() Coogan |
“The sheer amount of data we're getting — quickly we’re not going to be able to synthesize it on a human scale,” said Coogan, an assistant professor in both the School of Civil and Environmental Engineering and the School of Electrical and Computer Engineering.
His idea is to build the mathematical foundations for new models of traffic flow that process all of these new data streams and can offer new ways to control our transportation systems. Then, he’ll use techniques from computer science to prove that they work, so they’re guaranteed to deliver the results they predict.
“How can I provably, correctly say that the system is going to behave in a reasonable way? There's this huge gap between taking these techniques, which exist in this computer science world, and applying them to the physical systems that exist in the physical world,” Coogan said.
With the support of a National Science Foundation Early Career Development award, Coogan will spend the next five years working to bridge that gap, applying what computer scientists call formal methods to transportation network control systems. Traditionally, these methods are used to verify that a piece of software or hardware works correctly. Coogan will use them to prove his new models work.
He learned in January he had won the grant, known as a CAREER award and considered NSF’s most-prestigious funding for early career faculty. The CAREER program identifies potential leaders and academic role models, giving them funds to build the foundation for a lifetime of study.
“If we can break this code,” he said,” there's a lot of promise to have automatic deployment of algorithms and techniques to physical control systems.”
“There's a fundamental underlying mathematics driven by the physics here. Having the right models for this, being able to say something guaranteed — it's important, because it takes the human subjectivity out of traffic control decisions.”
The addition of autonomous cars to our roads offers one sample of the complexity Coogan must tackle. He said these cars will be able to follow each other very closely on highways, for example, traveling in what engineers call platoons.
“If we do that, back of the envelope calculations tell you that you can get three times the throughput on a freeway if every car on the freeway is autonomous,” Coogan said.
“But here's the question: Now you have not 100 percent autonomous cars [on the highway], you have 10 percent autonomy or 20 percent autonomy. You have some vehicles capable of platooning and regular, manually driven cars. How should we expect the traffic flow profile to change? … That needs a model.”
Coogan said that also complicates how we route autonomous cars versus human-driven cars so we get the most out of our road network.
“Google Maps already faces a pretty complex task trying to tell cars where to go,” he said. “Now, if you have to add into this mix how the type of car you’re driving is going to change traffic, then it becomes an even more complex problem.”
One of the new sources of data Coogan will use come from a partnership with a company called Sensys Networks, which uses sensors on roadways to detect every vehicle that goes by. Those sensors provide a level of detail down to the millisecond, he said, and show congestion forming and dissipating in real time. Seeing how all of those vehicle streams interact will help him understand how to do the fundamental math and build models of traffic networks.
Coogan said the work he does on this project also will build foundational theories that can be applied to other infrastructure systems.
“The underlying theme is that these are complex networks where we might have a decent sense of how the individual components behave, but you link them all together and you hit play, and then suddenly you get complex, nonlinear interactions between all the components in this system,” he said. “It's not enough just to be able to watch the data kind of flow past you and say, OK, let's try to make sense of the data.
“It’s much better to look at the fundamentals, the underlying models here, the underlying physics, and be able to predict how these complex networks are going to evolve over time.”